The project is still half completed and I put the summary introduction here, hopefully you guys like it and complete it with us :)
The structure of the robot is highly expandable, and it has high moving speed and environmental adaptability which are not available to the existing legged robots and wheeled robots through rolling motion.
The non-invasive BCI multi-functional rolling robot take advantages of BCI(Brain-Computer Interface) technology, which does not depends on the normal communication system consisting of peripheral nerve and muscle output pathways and can directly detect signal from brain activities. It also involves neuroscience, signals Detection, signal processing, pattern recognition and other interdisciplinary technologies.
The inference set come out from BCI is processed by Raspberry Pi 4b AR wearable device equipped with its own Python front and back end programs. With the collaboration of portable AR device and Bluetooth model , user could Interact with the robot through the disk program, which is used for data visualization and give instructions to the computer through EEG in a more orderly way. In order to obtain more pure and accurate EEG signal,the ignal processing should include the following three parts: preprocessing , feature selection and extraction, and feature classification. In this paper, common spatial pattern (CSP) algorithm is used to extract features, and LDA (Latent Dirichlet Allocation) is used to classify and train features. Finally, the movement direction of users' fact imagination is obtained.
The application of BCI tech and rolling robot are not just limited in the campus, it also can be applied in many fields in the society. For example, the cooperation of BCI technology and MR allows players to get more immersion and other special experience. It also could be applied to the product tools to improve the efficiency. Just because this kind of tech could be widly used in the modern society, it deserves more time and energy to be consummated.
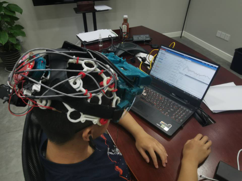
User wearing mixed reality and EEG headset “Ariel Supremacy”
2. OPENBCI
Different kinds of BCI signal collection tools have been developed while most are not affordable for teams with limited fund. The Openbci open-source board Cyton 8-
channel version was used in this project as a low cost solution and can be easily promoted to developer communities.
The Openbci also provides software supporting the interface between the Cyton board and computer, like Openbci GUI and Openbci Hub, allowing user to access and briefly process the signal collected, including using bias, controlling electrode states and frequency domain, which the Openbci GUI【2】 is able to interface with other application by LSL(Lab Streaming Layer) and other methods. Other BCI research applications like Openvibe【3】 and Neuropype【4】 are adaptable.
Fig. 3: Openbci GUI. Signal of electrodes of the user is observable.
3. METHODS
3.1 EEG
The EEG signal is a 5-100μv and low frequency bioelectric signal, which needs to be amplified before it can be displayed and processed. In the EEG signal processing and pattern recognition system, to correctly recognize the EEG signal, the signal processing should include the following three parts: pre-processing, feature selection and extracted feature classification. Signal pre-processing is mainly to remove low-frequency noise interference, such as using a spatial filter (CAR) to filter out low-frequency noise interference of ocular electricity and myoelectricity.
Feature extraction and selection are mainly for reducing the dimensionality of EEG data and extracting features related to classification.
At present, there are three main features of EEG data [5]: time-domain features, frequency-domain features, and spatial-domain features. Different features require different feature extraction methods. For...
Read more »